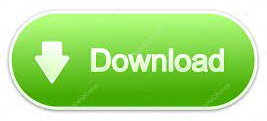

Therefore, this study aims to propose an efficient incident detection and classification (E-IDC) framework for smart cities, by incorporating the efficacy of model stacking, to classify the incidents with respect to their types and severity levels. This necessitates that the AID system detects and classifies not only all the popular traffic incident types, but severity as well that is associated with these incidents. While traveling along the road, one may come across different types of traffic incidents, such as accidents, congestion, and reckless driving. Most of the AID systems presented in the literature are incident type-specific, i.e., either they are designed for the detection of accident or congestion. Moreover, accurate classification of these incidents with respect to type and severity assists the Traffic Incident Management Systems (TIMSs) and stakeholders in devising better plans for incident site management and avoiding secondary incidents. Compared with the baseline model CapsNet, the model parameters of Res-CapsNet on dataset CIFAR-10 are reduced by 65.73%, while the classification accuracy is significantly improved by 33.66%.Īutomatic incident detection (AID) plays a vital role among all the safety-critical applications under the parasol of Intelligent Transportation Systems (ITSs) to provide timely information to passengers and other stakeholders (hospitals and rescue, police, and insurance departments) in smart cities. The experimental results show that Res-CapsNet has better performance on datasets, such as SVHN, FASH-MNIST and CIFAR-10. Res-CapsNet utilizes beta-mish activation function to reduce the "death" of neurons caused by ReLU, thus activating more neurons to further improve the classification accuracy.

Deconvolution reconstruction module is the last part of Res-CapsNet, responsible for reconstructing recognition results by using 4-layer deconvolution. Capsule module converts scalar neurons to vector neurons through the main capsule layer, and uses dynamic routing algorithm to selectively activate the high-level capsule in the main capsule layer and the digital capsule layer, and obtains the recognition results. The Res-CapsNet extracts deep features and sends them to capsule module using the dense connections of residual, which effectively strengthens the feature transfer and feature utilization.
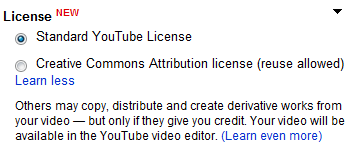
In this paper, a new residual capsule network model (Res-CapsNet) is proposed by fusing capsule network, residual network and deconvolution.
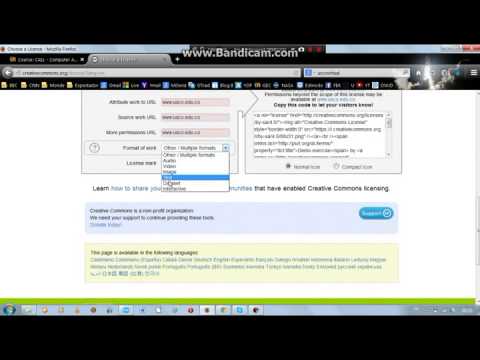
Capsule network can only extract shallow features, which makes it perform poorly on complex datasets. It overcomes the shortcomings of CNN that requiring large number of training samples and parameters, and information loss during the pooling process. Capsule network is a new network structure which can encode the properties and spatial relations of image features.
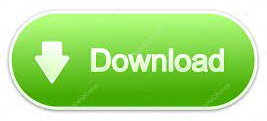